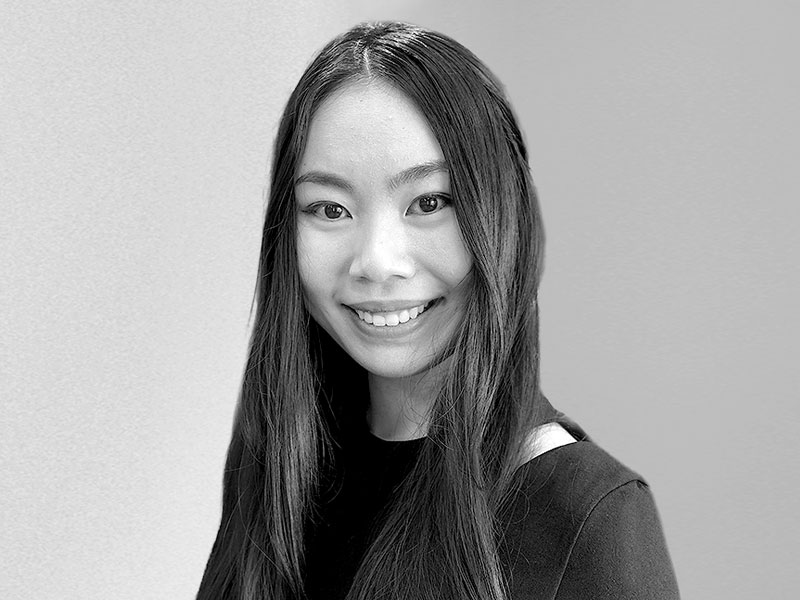
Jessica Hayes
Senior Associate
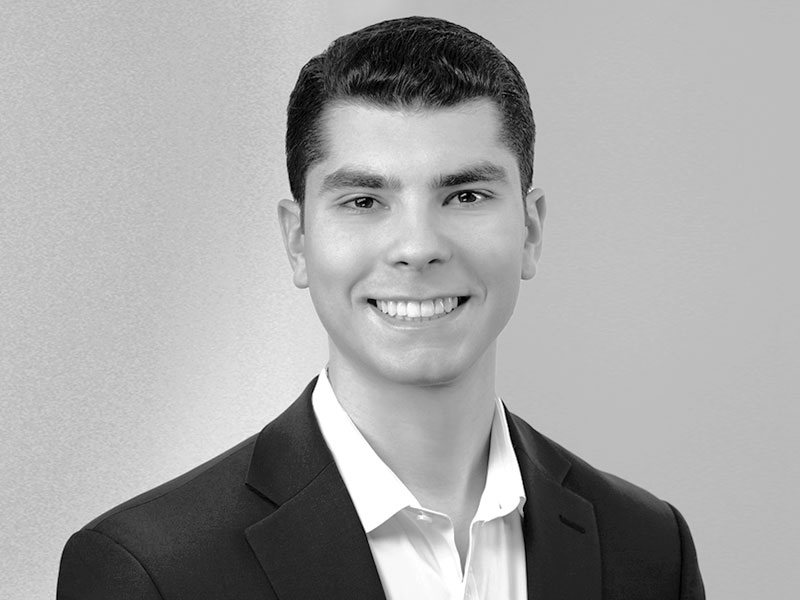
Ethan Volk
Associate
Part I
The AI Inflection Point:
Looking Back and Moving Forward
Over the past 30+ years, each decade has seemingly coincided with the evolution of a new core technological advancement. In the 1990s, we saw the internet digitally connect people across the globe; in 2006, Amazon launched AWS to begin the shift towards cloud computing; Apple debuted the iPhone in 2007, ushering in the mobile era of the 2010s. Now, in 2023, artificial intelligence (AI) appears to be the next disruptor which will define the course of markets, work and society for years to come.
Question 1: How Did We Get Here?
Artificial intelligence itself is not a new concept – in fact, AI in its earliest form is nearly 70 years old (the first AI proof of concept was published in the 1950s)1. Since then, artificial intelligence has continued to evolve. In the 1980s, convolutional neural networks (CNNs) were introduced to assist with object and character recognition, such as handwritten digits, zip codes and images. In 1992, the recurrent neural network (RNN) was developed to enable computers to process sequential data such as text (in 1997, a specific type of RNN called Long-Short Term Memory, or LSTM, was introduced as a potential solution to improve RNN’s ability to understand long-term dependencies). Over the past 10 years, there have been three major tailwinds that have led to the current state of AI.
1) Transformers: The Transformer model, which was introduced by Google in their 2017 paper “Attention is All You Need”, represented a significant advancement over previous models by emphasizing attention mechanisms in the model architecture. Specifically, the Transformer benefits from a type of attention called “self-attention” which allows the model to weigh the importance of different parts of an input sequence by comparing each part to all other parts of the same sequence in parallel, rather than processing the sequence sequentially. Transformer models improved upon RNN models by enabling more efficient and effective training and inference through the use of attention mechanisms and parallel processing capabilities, which allowed the model to process input data more efficiently and effectively. For example, Transformer models have outperformed RNNs in applications such as machine translation, language modeling and text generation.
2) Exponential Increase in Compute: Since 2012, the amount of compute used in large language models has increased at a pace far faster than Moore’s Law (which assumes a 2-year doubling period). For example, one model released in 2020 used 600,000 times more computing power than one of the earliest deep learning models released in 2012.2 This increase in computing power has directly allowed for larger, and often more accurate, models to exist.
3) Increased Creation of and Access to Data: Since 2017, a multitude of private and open source LLMs have been developed by companies including OpenAI, Google, Meta, Huggingface, A121 Labs, Cohere and Anthropic among others. While open source LLMs are publicly available, the cost of private API-accessible LLMs has continued to decrease (currently, OpenAI’s gpt-3.5-turbo is priced at $0.002 per 1,000 tokens), lowering the barriers to entry to build products using AI.
Question 2: Where Are We Now?
Since 2018, advancements in AI have accelerated at a rapid clip. With the Transformer enabling the creation of LLMs, companies large and small have rushed to publish their market-leading models. Just since March 2023, AI21 Labs released Jurassic-2 (178 billion parameters), Bloomberg released BloombergGPT (50 billion parameters) and Stability AI released StableLM (65 billion parameters). For the developer community, the opportunity set of models on which to build applications continues to increase.
AI partnerships with cloud giants have also formed. Most notably, Microsoft has aligned itself with OpenAI, investing nearly $13 billion in aggregate in the startup while incorporating OpenAI’s technology into its suite of products. Seeking to compete with Microsoft, Google made a $400 million strategic investment in competitor Anthropic in February 2023, giving Anthropic preferred access to its cloud infrastructure. Stability AI, which received $101 million in funding at a $1 billion post-money valuation in October 2022, announced its strategic alignment with AWS in November 2022 whereby Stability would leverage AWS’ compute infrastructure while also making its open-source models available on Amazon SageMaker JumpStart. By aligning themselves with the cloud giants, OpenAI, Anthropic and Stability AI gain exclusive access to best-in-class compute along with the capital required to train their models.
While building in AI has increased, so has investment. In 2017, global investment in AI was $21 billion; by 2019, it had increased to $38 billion (+81%). Global investment in AI peaked in 2021 at $108 billion amidst the broader tech market bubble before falling back to $69 billion in 2022.3
Taking a deeper look, the structure of funding rounds for US-based companies has evolved over the past few years4. In 2019, the median Series A and B entry valuations were $22mm and $65mm, respectively; as of 2022, the equivalent metrics doubled to $40mm and $148mm. At the same time, the amount of capital being raised per round has grown. Looking at Series A and Series B rounds once more, the median equity check raised increased by 33% and 40% since 2019, respectively. Valuations at the Series C round represented significant step-ups to the Series B. While the step-up between rounds lessened slightly in 2022 (2.0x), Series C companies received step-ups between 2.5x and 4.0x between 2019 and 2021.
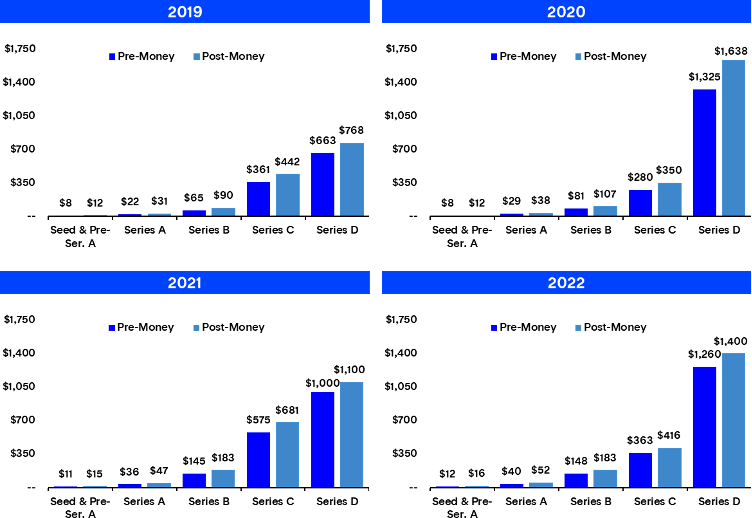
4
As AI permeates throughout the economy, several market research analysts are predicting AI to be a large driver of global GDP in the coming years (one such estimate suggests that AI will drive half of the incremental GDP over the next decade, representing 20% of global GDP in 20325). While the impact of AI on labor and jobs remains to be seen, we see many jobs and industries being partially exposed to automation via AI, resulting in workers being complemented by AI rather than completely substituted.
Question 3: What’s Next?
To accurately predict what the future of AI will look like is akin to throwing a dart at a dartboard situated on a moving vehicle 100 yards away – innovations are moving at a speed never before seen with new models and technologies developed every week. With that being said, there are a few overarching themes which we expect to drive future advancements in AI in the near term.
We expect AI models to be increasingly multimodal, or able to ingest and learn from data that comes in various modes such as text, image or video. Through a multimodal approach, AI models can create associations between different representations of the same concept (e.g., a picture of a dog and the word “dog”) to produce higher performing models. In March 2023, OpenAI announced that GPT-4 involved multimodality, though while it can ingest both text and images it can only respond via text.6
In addition to multimodality, we expect to see a focus on developing efficient AI models trained on smaller data sets which strive to match the performance of larger models. As LLMs increase in size – large-scale machine learning training models have prompted a 100x increase in demand for compute power7 – so too does their energy consumption. As a point of reference, GPT-3 took 1,287 MWh to train, or roughly the equivalent amount of energy required to power 120 US homes in a year.8
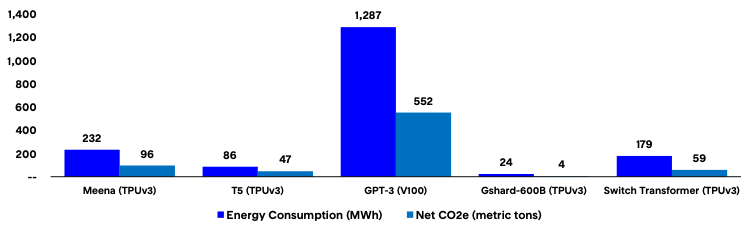
8
Moreover, with ESG and consumer data privacy concerns at the forefront of public discourse, an efficient AI model could shrink its carbon footprint while also decreasing the quantum of potentially sensitive data required to train it. ML researchers appear to be searching for novel ways to solve these problems – in February 2023, MIT researchers developed a technique that enables models to perform more reliably while using fewer computing resources and data than other methods.9
Building on the earlier discussion of responsible AI, we expect to see greater emphasis placed on explainable AI frameworks. AI models are often referred to as “black boxes” as the process by which an output is created is often difficult or impossible to explain. The ability to audit model algorithms will be important as growing emphasis is placed on ensuring that AI models promote fairness, account for bias and strive for accuracy. Should countries and governments pass AI regulations as many have started to discuss, we believe that explainable AI will be a core focus area.
We predict that AI copilots and personal assistants will become more widely adopted in the workplace, allowing for low-code / no-code usability by team members across functional areas. For software developers, companies including GitHub, Tabnine and HuggingFace / ServiceNow have released copilot products to assist in code generation and review, increasing productivity and reducing development costs.
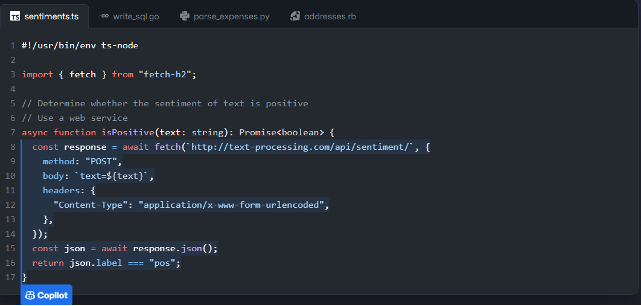
GitHub Copilot
Microsoft has already announced the integration of its Microsoft 365 Copilot solution into Office 365 applications while also debuting Business Chat, allowing users to ask work-related questions in natural language (e.g., “Can you summarize my email traffic from this morning?”). Moving forward, business leaders will seek to integrate these technologies into their organizations to boost overall productivity – according to a report by Goldman Sachs, generative AI could raise annual US labor productivity growth by nearly 1.5% over a 10-year period.10
Lastly, we expect to see an acceleration in smart software, or B2B SaaS solutions and platforms that are “leveling up” their offering by integrating generative AI capabilities into their product suite. Some of the world’s largest software companies have begun their generative AI journey. In March, Salesforce launched Einstein GPT to augment its customer relationship management product suite with AI; in April, Atlassian launched its Atlassian Intelligence product to improve service and project-based work for teams.
Interestingly, both Salesforce and Atlassian partnered with OpenAI to achieve their generative AI goals. While OpenAI has seemingly taken the early lead through ChatGPT, Google announced plans to integrate artificial intelligence into its products and services at its annual Google I/O developer conference in early May. From Search to Translate to Maps to Photos, Google plans to enhance its suite of products through generative AI. How and when B2B SaaS companies go about integrating AI into their products will vary, however, we believe that the leading B2B SaaS companies of tomorrow are either starting or are already down the path of becoming AI-first today.
Part II
Decoding the AI Investment Landscape:
Insights from AVP
As the current wave of AI innovation continues to take shape, we are looking forward to reaffirming our partnership with top-tier teams building best-in-class solutions powering or powered by AI. As investors, we are actively tracking four broad segments of the AI market landscape along with various sub-segments therein; moreover, when evaluating potential investment candidates, there are several factors that we believe are important to take into consideration.
Question 4: What Are We Most Excited About?
1) Horizontal Applications
Horizontal AI solutions benefit from applicability across industries – whether it be search, sales and marketing, customer experience or workflow automation, horizontal AI solutions can transform traditional business processes and departments through a data-driven and AI-first approach. At AVP, we’ve made two horizontal AI investments to date.
Phenom People provides an AI-powered talent relationship management platform, helping companies hire faster, develop better and retain employees longer. We led Phenom’s Series B and were impressed by the company’s ability to provide automated insights to recruiters filling roles across a variety of industries, resulting in a faster time-to-hire as well as an increase in application and conversion rate. In April 2023, the company introduced Phenom X+ which leverages an ensemble of AI models to bring generative AI to talent acquisition and management.
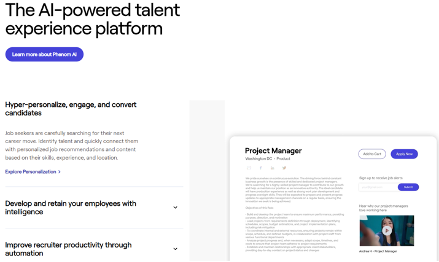
Phenom
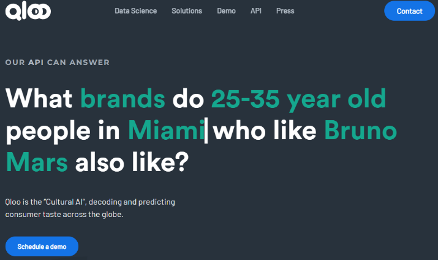
Qloo
Qloo provides a cultural AI platform that analyzes customer preference data to provide insights and recommendations for media, technology, CPG and hospitality brands. We were excited to lead Qloo’s Series B round due to the company’s deep dataset across several domains and ability to power sales conversion and sales efficiency compared to more costly methods of survey and focus groups, while remaining compliant with strict regulations around personal consumer data.
Segment Spotlight: Search
Search generates billions of dollars in revenue globally – in 2022, Google Search alone generated over $160 billion in revenue. Historically, search has been lexical, or based on the literal matching of words or phrases within an input query. While this approach has proved simple and largely effective, lexical search lacks the ability to understand the underlying meaning and context behind words. Conversely, semantic search takes into account the relationships between words and phrases, making it a more accurate and useful approach.
Semantic search is made possible by NLP models which can understand the context and meaning of text inputs and translate them into numerical vector representations, known as “vector embeddings”. These embeddings can be plotted on a graph in a vector database, allowing for the calculation of the distance (or semantic relationship, with smaller distances equating to higher similarity) between embeddings and produce the most relevant output based on an input query11.
Additionally, vector databases benefit from the ability to process unstructured data, saving time and effort required to review and prepare data for ML models. From an enterprise applicability perspective, we see tremendous value in the ability to ingest a corpus of internal documents and data, translate the text therein into vector embeddings and provide a user interface on top that allows for seamless vector search across and within documents.
2) Verticalized Solutions
Companies building vertical AI solutions focus on developing models and applications for use cases within specialized sectors including healthcare, retail, financial services, defense, logistics and legal. Though each market vertical covers a narrower scope, vertical AI companies have the benefit of being purpose-built for a specific industry, addressing explicit customer needs and concerns, often resulting in a stickier, “must-have” product.
Our portfolio company Incepto provides a platform that offers doctors and hospitals access to a portfolio of AI solutions, designed to improve the quality of diagnoses and save time for medical teams without having to change equipment. We led Incepto’s Series A round with our enthusiasm stemming from the prospect of improving health outcomes and mitigating the lack of specialists for certain conditions by distributing the most powerful AI tools for each specialty and creating new AI applications that meet real needs through co-development.
Segment Spotlight: Healthcare
We remain excited about healthcare, as we believe AI will allow for much more personalized care and bring a revolution in the use of large medical datasets. We’ll see patient-specific treatments — for example, treatments created using your own genomic and expression data which may lead to much more successful outcomes. AI should also help to accelerate the viability of continuous, remote-patient monitoring – with continued adoption of health-centric wearables, AI can identify troubling trends in data, enabling doctors to proactively treat their patients before it’s too late.
From a workflow perspective, material partnerships have formed – in April, Microsoft and Epic announced that Azure’s OpenAI Service would be integrated with Epic’s EHR software, enabling organizations to run Epic environments on the Microsoft Azure cloud platform and benefit from Microsoft’s generative AI capabilities.112 Nuance Communications (owned by Microsoft) also announced it would be integrating GPT-4 into its clinical notetaking software in March 2023 (roughly 77% of U.S. hospitals use Nuance’s technology).13
“Our exploration of OpenAI’s GPT-4 has shown the potential to increase the power and accessibility of self-service reporting through SlicerDicer, making it easier for healthcare organizations to identify operational improvements, including ways to reduce costs and to find answers to questions locally and in a broader context.”
SVP of R&D, Epic
Data and patient privacy will be a key concern in healthcare – to produce results of value, algorithms will need to be trained on enough relevant medical data. Synthetic data may help to solve the issue of maintaining patient data privacy, however, comprehensive security measures will be required to ensure that patient data does not fall into the wrong hands.
3) Generative AI
While traditional AI models are trained on mass quantities of data to identify patterns and provide insights, generative AI differs by producing net new outputs of various forms, including text, images, video and music based on input prompts. Over the past two years, there has been broad-based exuberance around the possibilities of generative AI. Whether it be for code completion, marketing content, video editing or text-to-speech, we believe the most successful generative AI companies will be those that prove to be “need to haves” rather than “nice to haves” through increased efficiency and clear, positive ROI.
Our portfolio company D-ID uses AI and deep learning to develop reenactment-based products ranging from animating still photos to producing high-quality digital avatars. We led D-ID’s Series A round and were excited by the large market opportunity, strong market tailwinds and the company’s proprietary technology. D-ID provides value to companies by diminishing the time and costs involved in video production in an ethical way, allowing for the creation of highly personalized media for e-learning, corporate training and marketing communications.
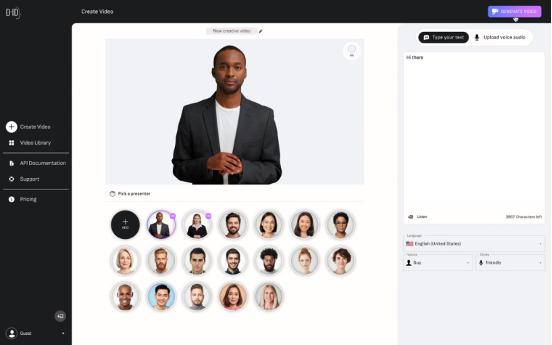
D-ID
Segment Spotlight: Enterprise Workflow Productivity
In a study conducted by Salesforce in March 2023, 57% of senior IT leaders responded saying that generative AI is a game-changing technology with the potential to improve customer experience, enhance data use and increase productivity.4 The vast majority of knowledge workers (94% according to Zapier) today spend time during their days working through repetitive and time-intensive tasks such as notetaking, data entry and file management. Moreover, U.S. productivity dipped 2.7% in Q1 2023 compared to 2022.15
With generative AI tools, tedious business processes can be automated, providing leverage to workers to focus on the parts of their job that drive the most value. We see a world where generative AI allows three-hour video calls to be summarized in seconds by several AI-generated bullets, bespoke sales outreach emails to be crafted based on a single prompt line and legal documents to be edited and redlined based on an organization’s requirements. We do, however, expect to see many of these generative AI solutions providing a complementary offering to knowledge workers as opposed to outright replacing them in their role.
4) Developer Tools and AI / ML Ops
To achieve everything described above in the horizontal, vertical and generative AI segments, a suite of platforms and tools must exist to build, train, deploy and monitor AI systems. Companies in this segment enable the full AI life cycle, from data collection and preparation, to model training and testing, to deployment and monitoring. There are many solutions emerging in areas such as model operations, data quality & observability, labeling and synthetic data. We see three approaches in this segment – i.) cloud vendors offering an end-to-end, cloud-native solution, ii.) hybrid platforms offering an end-to-end on-prem and cloud solution and iii.) point solutions addressing paint points in a specific area.
This is a relatively nascent space, with best practices evolving at a fast pace. The market is growing rapidly as more companies look to utilize AI, with many open source and managed solutions emerging. While AVP has not yet made an investment in this space, we are excited to partner with companies enabling the acceleration of AI with a differentiated approach. We have been excited about trends towards enabling developers to utilize data science tools, security and governance tools and tools improving the success rate of projects.
Segment Spotlight: Data Operations Tools
85% of AI projects will fail to generate their intended results due to bias in data, algorithms or the teams responsible for managing them16. Given the opportunity cost of time and capital spent on these projects, there has been a greater focus on data and testing operations.
At the earliest stages, enterprises deploying models need to ensure quantity and quality of data. On the quantity side, tools can assist in identifying and collecting relevant data from external and internal sets and can include text, video, and images. On the quality side, tools can help normalize and format data, while data annotation is still a relatively manual process. Data governance (such as security and privacy) and monitoring (such as data updates) are also crucial in data operations.
We are excited by the trend towards data visualization, whereby users monitor patterns in data before models are built to determine the most important parameters and model feasibility. Overall, we believe there will be a shift towards more observability and testing earlier in the life cycle to ensure models will perform in later stages of production.
Question 5: What Are Investors Looking for in AI Companies?
Every AI company is different – while there is no cookie-cutter approach to investing in the next generation of AI-powered technologies, there are several factors that investors should consider outside of the market, technology and team.
1) Access to Proprietary Data
While LLMs are pre-trained models trained on billions of parameters, they are inherently general-purpose models. For a vertical application – such as a SaaS platform that uses AI to monitor financial transactions specifically for AML – fine-tuning a LLM using proprietary data can lead to higher accuracy for a specific vertical use case. In this example, an AML SaaS company may have access to billions of transactions that would be used to train a model to be hyper-focused on money laundering.
2) Data Feedback Loops
In addition to proprietary data itself, we expect the best AI-driven companies to benefit from data feedback loops. LLMs can benefit from Reinforcement Learning from Human Feedback (RLHF), or the concept that models can improve based on positive feedback received from human interaction. Through feedback loops, AI systems can better understand user behavior and learn right from wrong, allowing for more accurate outcomes in the future. Companies which can not only capture data from human interactions with their product but also regularly feed the data back into the training set to fine-tune their models will ultimately be primed to succeed.
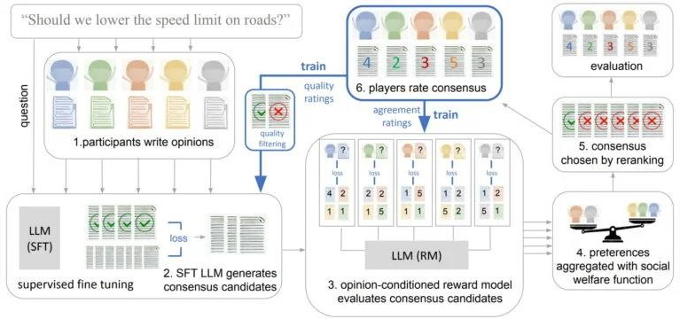
17
3) Product & Customer Experience
With access to AI models becoming increasingly accessible via open-source software and closed APIs, we expect to see more emphasis placed on providing a differentiated product and customer experience that precisely solves a specific customer problem. Moreover, especially in consumer-facing solutions, an intuitive front-end experience will be critical. OpenAI’s ChatGPT was able to reach one million users in five days not only because of its innovative technology, but also because of its simple and familiar chatbot interface.
4) Quantifiable ROI
AI has broad applicability across industries and use cases, from healthcare to sales & marketing to cybersecurity. With enterprise-focused applications, quantifiable ROI will be critically important – solutions which explicitly reduce operating costs, increase revenue or boost efficiency and accuracy will stand out from the pack of “nice to have” options.
5) Platform Interoperability and Integrations
The rise of applications being built on top of LLMs shares some similarities to the rise of cloud computing. In the same way that companies can choose a single cloud provider or opt for a multi-cloud solution, AI-powered applications can choose whether to build on top of OpenAI, Google, Huggingface, AI21 Labs or any one of the other closed or open-source models. We believe that a multi-LLM or even LLM-agnostic approach may allow companies to be more flexible, easily shifting between models as needed. Additionally, solutions that can easily be embedded into existing applications – such as a travel website or accounting system – will provide companies with plug-and-play capabilities to instantly level up their offering with AI.
6) Speed of Iteration and Updates
Over the past ten years, the amount of compute used in AI models has increased at an exponential rate. With that, models have gotten significantly better in a very short period (for example, OpenAI’s GPT-2 was released in February 2019 with 1.5 billion parameters; GPT-3 was released 15 months later with 175 billion parameters). Larger models do not always equate to better performance, however, companies will need to keep up with the newest technological developments, whether that be building on top of more powerful and accurate models or employing new AI development and deployment techniques, to maintain a market-leading solution. Ultimately, what might be deemed as technologically obsolete may occur quicker than it has historically – the ability for companies to be nimble and adaptable will prove to be of value.
7) Responsible AI
AI models are not immune from problematic outcomes – for example, Microsoft’s AI chatbot, Tay, was shut down after 24 hours after it began producing hateful and racist language in 2016. Shortly thereafter in 2017, Microsoft established Aether, an internal ethics advisory board which advises senior leadership on AI issues and best practices, specifically with the focus of building AI in a transparent, fair, inclusive and secure way. With increased public and governmental scrutiny, we believe it will be critical that all AI companies build and deploy AI with a strategy around responsible AI practices.
Parting Thoughts
We are still very much in the early innings for AI – with every passing month, we are amazed by the innovation that is taking place. As investors, we are excited to continue our partnership with some of the leading companies driving innovation within AI over the coming years.
If you’re an early stage or growth stage company actively building within the space, we would welcome the opportunity to speak with you.
You can connect with us at ethan.volk@axavp.com and jessica.hayes@axavp.com,
along with the rest of our colleagues at avpcap.com.
References
1 Harvard University.
2 “AI and Compute”, Georgetown University.
3 Pitchbook – Artificial Intelligence and Machine Learning Market Vertical.
4 Pitchbook screen includes United States based companies who received an equity investment of at least $1mm from January 2019 through December 2022. Incudes all investments received by a company during the period, not just the most recent investment. Does not include companies who did not disclose the amount raised or any details on valuation. Figures (in millions) represent medians.
5 Oppenheimer Equity Research, March 2023.
6 The Verge, “OpenAI announces GPT-4 – the next generation of its AI language model”.
7 Oppenheimer Equity Research, March 2023.
8 Patterson et al., “Carbon Emissions and Large Neural Network Training”, April 2021. Chart measures Energy Consumption and CO2e for five large NLP models.
9 MIT, “Efficient Technique improves machine-learning models’ reliability”.
10 Goldman Sachs Equity Research, March 2023
11 VentureBeat.
12 Microsoft.
13 Healthcare Dive.
14 Salesforce.
15 Ernst & Young.
16 Gartner.
17 DeepMind.